Algorithmic Trading Demystified: A Beginner’s Guide to Automated Success
- Ross Hidge
- Sep 6, 2024
- 2 min read
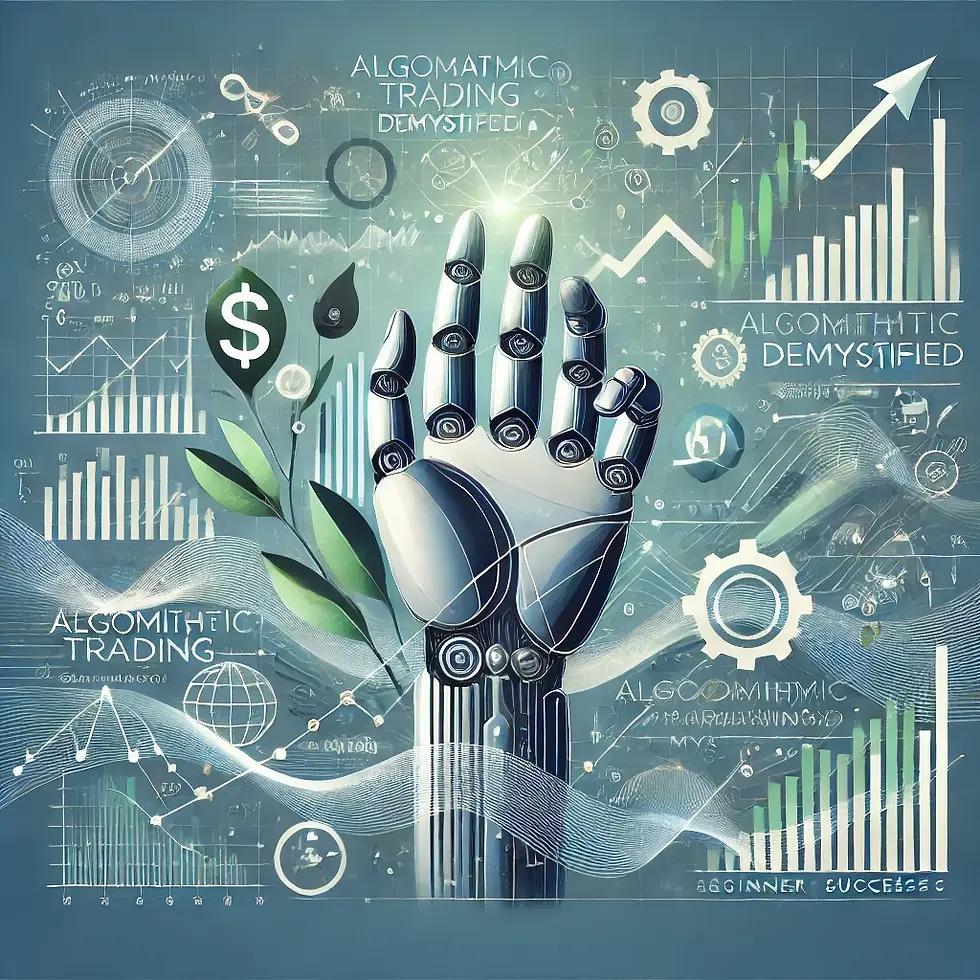
In the fast-paced world of finance, algorithmic trading has emerged as a game-changer, offering a way to execute trades with precision and speed far beyond human capabilities. If you're new to this concept, you're not alone. Let's demystify algorithmic trading and explore how it can pave the way to automated success.
What is Algorithmic Trading?
At its core, algorithmic trading involves using computer algorithms to execute trades based on predefined criteria. These criteria can include various factors such as price, volume, and time. Algorithms analyze market conditions and make split-second decisions, often executing trades faster than any human trader could.
Why Algorithmic Trading?
Speed and Efficiency: Algorithms can process vast amounts of data and execute trades in milliseconds, ensuring that opportunities are not missed due to delays.
Elimination of Emotional Bias: By adhering to predefined rules, algorithmic trading removes emotional decision-making from the equation, leading to more disciplined trading.
Backtesting and Optimization: Algorithms can be backtested using historical data to refine strategies before applying them in live markets.
Key Components of Algorithmic Trading
Strategies: At the heart of algorithmic trading are strategies—rules that dictate how trades should be executed. These strategies can range from simple moving average crossovers to complex machine learning models.
Data: High-quality data is crucial for making informed trading decisions. Algorithms rely on real-time and historical data to identify patterns and make predictions.
Execution: The execution system ensures that trades are placed accurately and promptly according to the algorithm’s rules.
Types of Algorithmic Strategies
Trend Following: This strategy aims to capture the momentum of a stock or asset by buying when the trend is up and selling when the trend is down. Common indicators include Moving Averages (MA) and Relative Strength Index (RSI).
Mean Reversion: Mean reversion strategies assume that asset prices will revert to their historical mean. Indicators such as Bollinger Bands and the moving average convergence divergence (MACD) are often used.
Arbitrage: This involves exploiting price differences between related assets or markets. Arbitrage strategies require real-time data and quick execution to capitalize on fleeting opportunities.
Machine Learning: Advanced algorithms use machine learning models to predict market movements based on historical data and patterns. These models can adapt and evolve over time, potentially improving their accuracy and effectiveness.
For those new to algorithmic trading, it can be beneficial to start with a user-friendly platform that offers pre-designed strategies and an intuitive strategy builder. Tools like StratifyPro are designed to simplify this process, providing users with a visual strategy builder, extensive backtesting capabilities, and a library of pre-designed strategies. With features such as zero-code strategy creation and API connections to brokerages, StratifyPro makes it easier for beginners to start automating their trading strategies.
Algorithmic trading represents a significant advancement in how trades are executed and strategies are developed. By leveraging algorithms, traders can enhance their efficiency, minimize emotional biases, and potentially achieve better results. For those looking to dive into the world of automated trading, tools like StratifyPro offer a streamlined approach to harnessing the power of algorithms, making it easier to get started and succeed in the market.
Comments